Research Projects in Data-Enabled Industrial Mathematics
ERAU Research Experience for Undergraduates
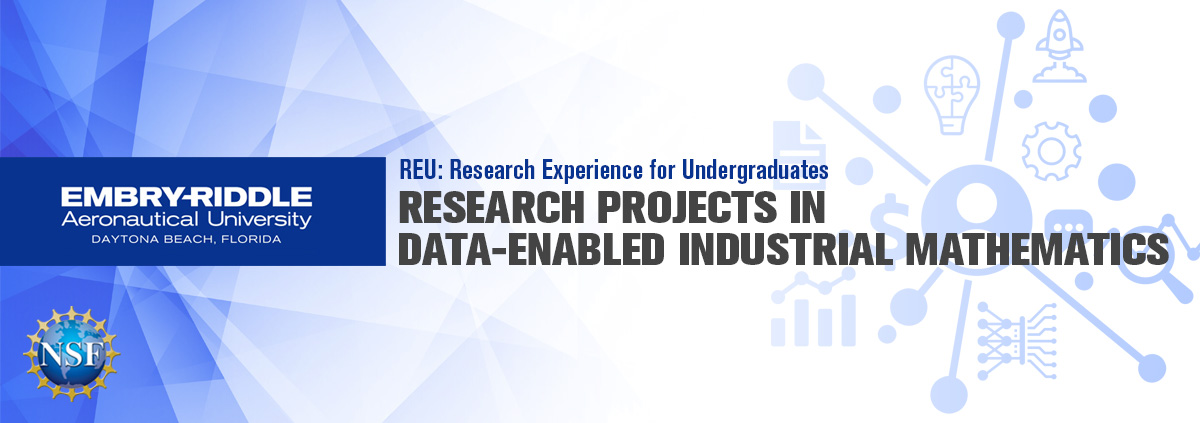
Summer 2023 REU
INDUSTRIAL PARTNERS
![]() |
![]() |
|
![]() |
Nevada National Security Site, Las Vegas, NV | Pacific Northwest National Laboratory, Richland, WA | FlexJet, Daytona Beach, FL | Brave Enterprises, NYC, NY |
Projects
Quantifying Uncertanty in Segmations of Computed Tomography
Industrial Sponsor: Pacific Northwest National Laboratory, Richland, WA
Description and resultsStudents: ERAU REU: Research Projects in Data-Enabled Industrial Mathematics (Summer 2023) Ellie Kienast ('24) - Georgia Institute of Technology - Mathematics Nathaniel Reimer ('24) - Macalester College - Data Science Hadley Santos-Del Villar ('24) - SUNY University at Albany - Informatics Yianni Paraschos ('24)- ERAU - Aerospace Engineering and Computational Mathematics Description: Image processing and analysis play pivotal roles in materials science, manufacturing, and non-destructive testing. By processing computed tomography (CT) images, researchers can derive physical characteristics of an object, such as its boundary, surface, and internal features. Identifying different areas of an image and the edges between them is known as segmentation. Pacific Northwest National Laboratory (PNNL) utilizes CT scans to study objects and materials in an efficient and nondestructive manner. Partnering with PNNL, this Research Experience for Undergraduates project at Embry-Riddle Aeronautical University aims to quantify the uncertainty of edge detection methods applied to CT scans of machined objects. To ensure the reliability and effectiveness of CT, the associated uncertainty in segmentation must be addressed. The first phase in our research applies gradient, statistical, and area-based edge detection methods to a dataset of 176 CT images comprising a single test object provided by PNNL. Evaluation metrics are employed on each edge detection method, and a ground truth reference is generated to determine the accuracy of the algorithms. After selecting the most effective method, frameworks for quantifying error in edge detection are developed, tested, and evaluated. Using the developed error models as a basis, the uncertainty of the CT scan segmentation can be quantified to generate a more robust and productive process to study materials in industry application. Major outcomes:
|
Uncertanty Propagation in Image Deblurring
Industrial Sponsor: Nevada National Security Site, Las Vegas, NV
Description and resultsStudents: Eleanor Sigel ('25) - University of Southern California - Applied Math Madeline Gorman ('24) - ERAU - Computational Mathematics Thomas Pasfiled ('25) - ERAU - Aerospace Engineering Description: Image blur poses a significant challenge in radiographic data analysis, hindering re- searchers’ ability to interpret critical information accurately. Regularization methods, such as Tikhonov (L2) Regularization and Total Variation (R.O.F.) Regularization, have emerged as ef- fective tools for image deblurring. A Point Spread Function (PSF) is used to estimate the blur on an image without knowing the cause. However, these methods introduce uncertainties into the estimation process, which can impact the accuracy and reliability of the deblurred images. In this article, we present a comprehensive analysis of uncertainty propagation in image deblurring and investigate the effects of Tikhonov and Total Variation Regularization on blur estimation. By quantifying uncertainty and analyzing error bounds, we gain insights into the limitations and trade-offs of each method. The findings contribute to improving the accuracy and reliability of image deblurring techniques and advance the field of image processing. Major outcomes:
|
Spatio-Temporal Analysis for Modeling High-Demand Events in European Private Aviation Travel
Industrial Sponsor:FlexJet, Daytona Beach, FL
Description and resultsStudents: Murphy John ('24) - University of New Mexico - Applied Mathematics and Statistics Samantha Mackley ('24) - University of Missouri - Economics and Statistics Nicole Morgen ('24) - Carroll College - Mathematics Michael Leitelt ('24) - Stetson University - Financial Mathematics Noa Teed ('24) - ERAU - Software Engineering Talia Foley('24) - Grinnell College - Mathematics Description: Accurately predicting the demand for aviation is a complex problem that is essential for the success of the private aviation providers. Factors such as seasonality and location affect the demand for private flights, but high-demand events and holidays introduce additional and often unexpected influences on these services. In European destinations, travel is heavily characterized by high-demand events and holidays. This research utilizes detailed characterization data centered in Europe containing over 1.1 million private flights between 2,016 locations from 2018 and 2019. Leveraging advanced data analysis techniques, this project constructs a spatio-temporal forecasting model to accurately predict the demand for private jet travel during high-demand events and holidays in European destinations. This research delivers valuable insights to providers of private aviation, enabling them to proactively respond to market fluctuations and optimize their operational strategies. Major outcomes:
|
Essential contributing factors of bravery
Industrial Sponsor:Brave Enterprises, NYC, NY
Description and resultsStudents: Kylie Loftis ('24) - Virginia Commonwealth University - Statistics Citlali Rocha-Ruiz ('24) - Kansas State University - Mathematics Description: Brave Enterprises is a company that designs and implements dynamic training that enables people to recognize fear as a cue to take brave action. Part of the training sessions, requires participants to complete a pre-survey prior to the 2-hour session and a post-survey following the session. Embry-Riddle REU students were given the opportunity to research and analyze various datasets provided by Brave. This analysis used more than a thousand matched surveys from various sessions done by Brave Enterprises since their founding in 2016. Our goal was to identify what factors lead to a person’s sense of bravery. The advanced analysis performed included: feature importance, cross correlations that lead to high bravery scores, and efficiency of the program. It was expected that leadership style, choice of role model, and sense of purpose were strong indictors of one’s bravery score. By understanding which factors are the most predictive, regarding bravery score, we can contribute and support Brave’s mission of helping people tackle their obstacles, grow confidence, and be more brave. Major outcomes:
|
Students
![]() |
![]() |
![]() |
Talia Foley ('24) Grinnell College Mathematics | Murphy John ('24) University of New MexicoApplied Mathematics and Statistics | Ellie Kienast ('24) Georgia Institute of Technology Mathematics |
![]() |
![]() |
![]() |
Michael Leitelt ('24) Stetson University Financial Mathematics | Kylie Loftis (‘24) Virginia Commonwealth University Statistics | Samantha Mackley ('24) University of Missouri Economics and Statistics |
![]() |
![]() |
![]() |
Nicole Morgen ('24) Carroll College Mathematics | Nathaniel Reimer ('24) Macalester College Data Science | Citlali Rocha-Ruiz ('24) Kansas State University Mathematics |
![]() |
![]() |
![]() |
Hadley Santos-Del Villar ('24) SUNY University at Albany Informatics | Eleanor Sigel ('25) University of Southern California Applied Math | Madeline Gorman ('24) ERAU Computational Mathematic |
![]() |
![]() |
![]() |
Ioannis Paraschos ('24) ERAU Aerospace Engineering and Computational Mathematic | Thomas Pasfield ('25) ERAU Aerospace Engineering | ERAU Software Engineering |
CONTACT INFORMATION
Please contact REU site coordinator Dr. Berezovski at berezovm@erau.edu for any inquiry about REU Site and application process.